First published 12 Oct 2019 in LinkedIn
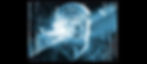
Everyone’s doing AI. Is this another case of teenage sex where everyone thinks everyone else is doing it, but nobody’s doing it properly?
Just about everyone on the street was talking innovation but few knew how to nurture it, let alone derive sustainable benefit from a robust incubator framework.
Same applies to AI. Let me break this down.
What is artificial intelligence?
As in real life, intelligence levels has many tiers from the common ability to do things automatically, to being smart up to outright genius. We’re talking broad spectrum here.
Here’s my take to pare it all down, look at the big picture, what the enablers are and understand what it takes to spearhead an AI movement.
In computer science, artificial intelligence, sometimes called machine intelligence, is intelligence demonstrated by machines, in contrast to the natural intelligence displayed by humans. The practice of deriving benefits from deploying artificial intelligence is really sparked off by the maturing, democratisation and wide availability of machine learning algorithm, as a service. (A myriad of services are available from Amazon Web Service, Azure, Google Cloud, Alibaba Cloud and even Facebook – Pytorch Mobile).
Machine learning is the application of computer software codes, leveraging upon mathematical and statistical models to perform a task, relying on patterns and inference instead of depending on specific instructions. Machine learning “feeds” on data, and attempts to make predictions or decisions, “independently”.
The key question is, how many know how to turn AI practice to produce sustainable business benefits, combining short term wins with longer term road maps empowering the entire organisation
The key question is, how many know how to turn it into sustainable business benefits, combining short term wins with longer term road maps empowering the entire organisation?
Big picture – The AI ecosystem for the next decade, can be summarised in a hexagram below.
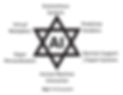
AI Ecosystem drill down
Autonomous Systems
Automation is not a new concept. What is new is the easy availability & affordability of new tools like UI Path, Blue Prism and Automation Anywhere (world first cloud native platform) to automate value chains (sometimes wrongly e.g. doing wrong things faster). These software fall under a sub-category of autonomous systems call Robotic Process Automation (RPA). Many leading corporations have already deployed RPA. Service organisations have replaced hundreds of call center staff with just a couple of RPA bots which when well implemented (within 6 months from inception to closure), delivers enviable ROI. Some have even used bots to assist in market price gauging activities, whilst others use it to perform repetitive back office tasks.
Service organisations have replaced hundreds of call center staff with just a couple of RPA bots which when well implemented, delivers enviable ROI
Bot applications are also being planned for even complex operational activities like watching over warranty for entire fleets of aircraft, sensing when a particular part is being removed every second of the time, cross checking against a warranty database or contractual agreement, then assigning a priority and queuing it to the most qualified officer. The “oracle” does it and learns who is more productive and even rearranges queue items depending on probability of claim success and priority status. RPA’s micro-second fidelity and 24 hours operations, are hard to beat and extremely cost effective.
Furthermore, elements in the hexagram doesn’t need to stand alone. RPA bots, for instance, can work with predictive algorithms in the case of aircraft warranty, to be able to predict likelihoods of warranty claim success and quantum of returns. In Automation Anywhere’s case, their offering can be integrated to third party computer vision, natural language processing and predictive modelling partners.
Predictive Analytics
Whilst most competent companies today have a good business intelligence and analytics capability, predictive analytics raises the bar further. Reporting has evolved from producing lagging indicators, to displaying real time progress and now, is embarking upon predicting the future.
Reporting has evolved from producing lagging indicators, to displaying real time progress and now, is embarking upon predicting the future.
That way, operational teams can be geared to focus on what matters most. Progressive airlines, road and rail transportation companies are already deploying and further refining predictive model on key facets of their operational performance, the better models pin point root causes accurately, for steering actions. This is where integration with Decision Support systems complete the loop to deliver business benefits
Expert Systems / Decision Support
Whilst traditional business intelligence produce insights at a basic level, expert systems, in conjunction with predictive analytics, are integrated with decision support systems tailored into workflow apps which then turn insights into benefits.
The quality of UI/UX interactivity can reform traditional linear / serial value chain into multiple parallel, high speed, hyper efficient operational outcomes.
Simplicity is key.
Those workflow apps, can be designed with company policies & procedures baked in, disrupting a multitudes of old world artifacts like policy and procedure manuals. Naturally, UI/ UX app design is paramount, as the quality of graphical interactivity can reform traditional linear / serial value chain into multiple parallel, high speed, hyper efficient operational outcomes. Simplicity is key!
A typical example is a workflow steering app can effectively handle complex operational disruptions when a train hits a person or a signal fault disrupts a particular section of a transportation network.
Human Machine Interaction
We all know about chat bots and virtual assistants. What is more important, is the realisation of the overall category of human machine interaction and how it can be deployed in unique business environments. Chat bots are largely textual input based (could be quickly disrupted by voice or other graphical UI methods), virtual assistants (utilising natural language processing) are largely audio based. Machines can listen then talk to you and increasingly, they can also see you, very well.
The superstar in this category, is undeniably machine vision. Machine vision has made headlines because it is one of the major front of the 2019 Sino-American technology war (dubiously labelled trade war). Americans led in research but it is the Chinese who is leading in implementation and recently outgunned everyone in patent filings. The top 5 machines vision champions in global competitions are repeatedly won by Chinese organisations, trumping all others in accuracy and detection speed. Whilst there’s debate about the ethics of implementation, machine vision can be implemented in a variety of ways. In comes the typical analogy, is a knife good or bad? Whilst the Chinese are being criticised for the way it was implemented, the Australians are already starting to plan for a nationwide facial recognition database.
Hyper Personalisation
Cheap processing power and powerful machine algorithms allow us to move from a mass production economic paradigm (one to many) into a mass customisation (many one to one) advantage. Learning each customer as an individual, via their search patterns and browsing habits helps back end AI to push customers down the sales funnel, turning a disinterested person, to a person with need, then converting that eventually into a successful transaction.
Learning each customer as an individual, via their search patterns and browsing habits helps back end AI push customers down the sales funnel, turning a disinterested person, to a person with need, then converting that eventually into a successful transaction.
Mass customisation is often a key jigsaw in growth hacking marketing strategies. Hyper personalisation is popular in industries such as finance, healthcare, or personalized fitness and wellness applications.
Virtual Multipliers
Another movement which is generating groundswell is the migration of value adding (starting from the service sectors) from the physical work to the virtual world. 3D worlds are evolving rapidly from the world of gaming to the corporate world. A day will come when all commercial offices will get disrupted.
In virtual reality, there are arguable 3 major classes of VR,
3D on 2D for e.g. the traditional “3D world” game second life, High Fidelity platform,
HoloLens 2 which is 3D superposed into actual reality by way of Microsoft HoloLens glasses (imagine a virtual prototype of a new engine valve sitting on your work desk in your discussion with an engineer in another country) and
Full-fledged VR where you are totally immersed in an VR goggle – this may cause disorientation after prolonged use and involves a relatively larger capital expenditure.
In the case of 3D on 2D, Professor Eddie Obeng in UK has for many years been successful in delivering great value via his amazing QUBE environment where programs and projects are delivered virtually. In his environment, laws of physics which impede us in the real world, are modified to enable significantly higher productivity. Arguably, a well-designed virtual delivery platform can outdo physical productivity / value-add by multiples of 2 to 8, for starters.
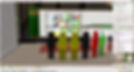
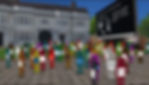
Fig 2: QUBE Virtual Collaboration World
In order to partake and reap the benefits of the AI Ecosystem, there are critical enablers which every C-suite / leadership team needs to prepare. Just as any robust building starts with strong foundations, the House of Enablers depict the key requirement for any AI venture to be sustainably successful.
House of Enablers Drill Down
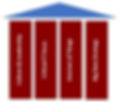
Fig 3: House Of Enablers
Culture & Leadership
Any organisation that rewards fire-fighting and fails to strategically plan forward, is like fish rotting from the head. From a Myers Briggs perspective (MBTI), has leadership and HR been rewarding only one particular character type, like the extroverts but ignoring the quietly thoughtful (but equally powerful) introverts? Or maybe the team had failed to check for bozo explosions (a manager hiring someone less intelligent and yes-men, and his subordinate does the same downwards . . gives rise to entire circus of clowns).
Another old cancer is recruitment policy of paying peanuts, and we wonder why they are so many monkeys?
If the culture is laid back and leadership does not play visionary roles empowering the organisation, with a high quality second tier management to back it up, it will be a budgetary disaster to embark on a half-arse journey (Joke: A half-a horse just goes in circles).
Critical-IT focus
An IT department that wants to do everything, in the end does nothing. The best lean IT departments will outsource commoditised offerings and manage it as a service whilst keeping differentiators close to its chest. A huge swathe of IT head count needs to be converted or replaced with different DNA, professionals who are experts in service management and savvy people-operation focused professionals able to integrate deeply and inspire operational departments with business savvy.
Experienced crack teams often leverage external contractors like Kaggle grand-masters and leading edge university research teams, because these people are of a different breed that will die off quickly if you put them in a permanent job cage.
There is a second group of AI, data science savvy chaps and niche crack dev-ops teams, pushing the boundaries of what great IT delivery looks like. Experienced crack teams often leverage external contractors like Kaggle grand-masters and leading edge university research teams, because these people are of a different breed that will die off quickly if you put them in a permanent job cage.
Buy off the shelf products, and you'll get off the shelf outcomes. That's why world beaters like Singapore Airlines goes half way round the world to engage the best professors from Stanford to do optimisation and form alliances with the best and brightest.
Gone are the days when IT mostly runs technical operations, maintaining virtual machines, service desk and email servers. The migration of traditional techies into outsourced companies and eventually into the cloud giants, have been happening for some time. If this trajectory remains, none of the outsourced companies will survive the cloud giants which will offer everything.
Internet Of Things – IOT
Way before IOT became a sexy term, forward looking companies were already exploring how we get non-human (non-subjective) data via sensors in our operating environment. Engineers will be familiar with strain gauges, RFIDs (active, passive systems), GPS telemetry and a breadth of physical measurement transducers available.
This, as we know has lately blossomed, together with unstructured data from cameras etc, into what we call Internet of Things, where those usually siloed applications are integrated into the big data’s data lake (as the name suggests, a relatively more unstructured consolidation of databases compared to a data warehouse).
We are also not short of enabling technologies to bring the data home, like Near Field Communications (NFC), Blue Tooth Low Energy (BTLE) with LoRa Mesh, Wi-Fi, ZigBee and the recently announced Amazon Sidewalk – a longer ranged low energy communications protocol.
Again, progressive organisations are already embarking on using empirical data from existing IOT devices like the black box / data logger on a passenger train, to derive insights to help in predictive maintenance, fuel savings and more.
Big Data Strategy
So we have heaps of data already present in traditional formats and added a few petabytes from IOT and marketing department’s Social Commerce strategy. How do we monetise it?
Big data is a discipline which presents ways to analyse, systematically extract information from, or otherwise deal with data sets that are too large or complex to be dealt with by traditional data-processing application software. A good big data strategy is able to find maximum value from the 4 Vs of big data.
o Volume – handling large amounts of data
o Variety – managing different forms of data
o Velocity – analysis of streaming data
o Veracity – managing uncertainty in data, not knowing how much is inaccurate
To get the basics right on existing data sets, many companies have embarked upon data integrity transformation programs. We all know the saying, Garbage in Garbage Out. Basic behaviours like ownership and data quality assurance has to be a subliminal habit in the organisation’s cultural fabric.
A high quality information strategy gives rise to a sound big data implementation. Quite a few organisations are sleepwalking without one, whilst those that’s blessed with dynamic leadership are striving to turn things around to build robust data lakes for their ace data scientists to play in.
In conclusion, the success of delivering an AI Ecosystem can vary over a wide spectrum, depending on the quality of human capital and the culture in which they are immersed in.
Leave your comments below, I would love to learn what your experiences are in this area.
#AI #digital #computing #Internet #bigdata #datalake #CIO #IT #informationtechnology #innovation #culture #business #disruption #predictiveanalytics #RPA #IOT #virtual #VR #machinevision #AIecosystem #data #datamining #DWH #datawarehouse #intelligent #intelligentorganisation #knoweldgeeconomy #knowledgeworker #futureofwork #future